
Research Projects
Research Project: Hydrological Modeling and Simulation
Background:
-
Precipitation is driving the water cycle and has exhibited extended variability and randomness over the globe
-
Watersheds are behaving differently due to changing external hydrological and climatological forcings.
-
It is essential for hydrologists to use models and analytical tools to further understand the physical dynamics of water-cycle and quantify accurate water-energy fluxes.
Approach:
-
Both conceptual and physical based hydrologic models are useful tools for simulating water cycles and mimicking the responses of watersheds.
-
Parameters usually need to be calibrated correspondingly for different watersheds or river basins driven by different observations for precipitation, evaporation, soil moisture, and streamflows.
-
We develop and apply different hydrological models and calibration methods to understand the complex hydrological responses in different regions over the United States and China
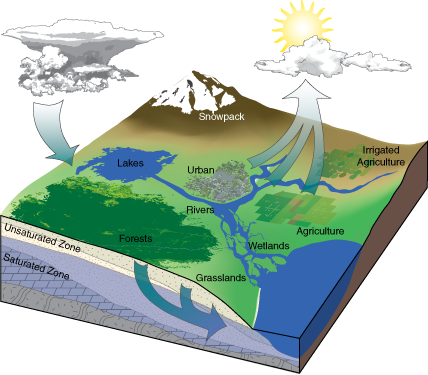

Figure. (left) water cycle and (right) schematic of the SAC-SMA model. (Source: Gan et al., 2014)
Research Project: Methods to Estimate Optimal Parameters for Hydrological Models
Background:
-
Model, data, and calibration methods are three fundamental elements in hydrologic process modeling and forecasting. Recent progress in hydrologic modeling has been made toward more efficient and effective estimation of model parameters.
-
We develop classical and advanced parameter optimization methods and study their applications in hydrological model calibration
Approach:
-
Both conceptual and physical based hydrologic models are useful in simulating water cycles and responses of watersheds.
-
Those methods include gradient-based optimization methods, direct search methods, and recent developed stochastic global optimization methods
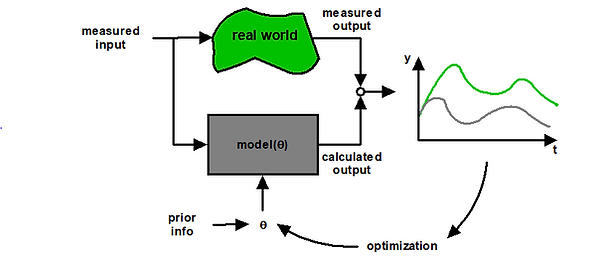
Figure. Strategy for model calibration​
Research Project: Sustainable Reservoir and Hydropower Operation and Modeling
Background:
-
A large number of hydropower dams have been built globally.
-
Non-stationary hydroclimates are altering fundamental engineering design and operation
-
A deeper understanding is required of how changing climate and varying weather conditions impact water-energy supplies, electric grid reliability, greenhouse gas implications, and ecosystem and social economic functioning.
Approach:
-
Use different scales of hydrometeorological forecasts to drive reservoir operation models and optimal hydropower scheduling strategies.
-
Develop Predictive reservoir simulation models, and predictive models from the state-of-the-art machine learning and data-mining techniques to support sustainable reservoir and hydropower modeling and decision-making
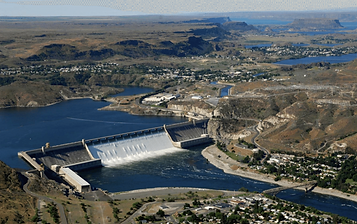
Figure. Grand Coulee Dam located on the Columbia River in the Washington State of U.S.A
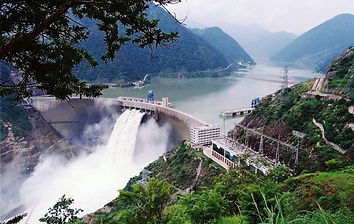
Figure. Ertan Dam located on the Ya-long River in the Sichuan Province of China.
Research Project: Climate Change and Evaluation of the Impacts on Water Resources
Background:
-
Climate has brought increasing temperature and caused different types of extremes
-
GCMs and Regional GCMs are the state-of-the-art tools in quantifying the impacts of climate and providing projections for future variabilities.
-
Uncertainties are associated with all individual GCMs and the reliability of model projections needs to be studied before practical uses.
Approach:
-
We develop multi-model ensembles methods to evaluate the reliability of climate model results.
-
We also compare different GCMs with ground observations to make suggestions for water resources managers on the accuracy of temperature and precipitation projections.
-
Evaluations of future water availability and climate extremes are also important from the management perspective in using GCMs.
-
High-mountainous areas are even challenging as the ground truth observations and in-situ gauge networks are not available. We develop alternative strategies in helping Himalaya and Tibetan Plateau communities in accessing data and better managing natural hazards.
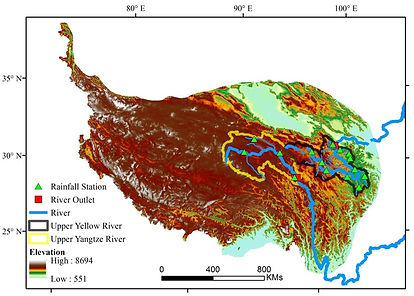
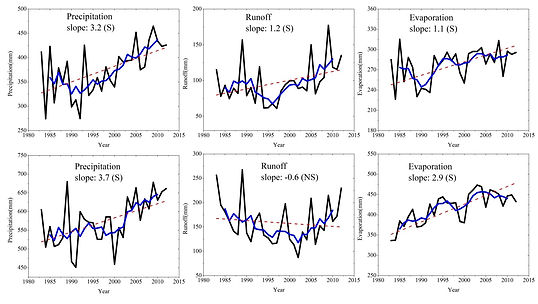
Figure. Left figure shows the Upper Yangtze River Basin (138,000 km2 on average of 4 Gauge station per 1°×3°), and the Upper Yellow River Basin (120,000 km2 on average of 11 Gauge station per 1°×1°). Right figure shows the tends of precipitation, runoff and evaporation over the Tibetan Plateau areas.
Research Project: Developing Advanced Optimization Tools and Algorithms for Water and Energy Resources Allocation
Background:
-
Many types of optimization tools have been developed over the last decades, including Genetic Algorithms, Particle Swarm Intelligence, Simulated Annealing, and many others.
-
It is essential to know the limitations and advantages of each algorithm when using those heuristic search algorithms in practice.
-
Effective and efficient optimization algorithms have great values in solving scientific problems and developing optimal solutions in many aspects of our life.
Approach:
-
Parallel computing techniques and clusters are used to develop a new hybrid, global optimization algorithms
-
The advantages and disadvantages of different types of heuristic search algorithms are revealed by a multi-threads competition mechanism in the newly developed algorithm

Figure. A newly developed hybrid global optimization algorithm which uses multi-threads competition strategy from various types of evolutionary algorithms
Research Project: Applying advanced artificial intelligence and data mining techniques to assist water resources management and planning
Background:
-
Artificial Intelligence and Data Mining (AI & DM) techniques have been rapidly developed over the past decade.
-
The usefulness of AI & DM does not restrict to computer science. The nature of water resources management and planning involves a great amount of data and uncertainties.
-
AI & DM techniques are suitable tools in assisting various tasks in the arena of water management, for instance, streamflow prediction, rainfall forecasts, reservoir release simulation, discharge rule retrieval, linking climate phenomenon to regional hydrology, and many other classification and prediction problems
Approach:
-
We develop advance simple Artifical Neural Network, Recurrent and Concurrent Neural Network, Deep-learning algorithms for data classification and prediction tasks.
-
Differs from the black box ANN models, Decision Tree models (as one of the white-box models) are transparent in its and easy for water manager to use. Research efforts are made on how to increase the accuracy and effectiveness of different decision tree models and applying advanced decision tree models to assist reservoir operation for better decision making.

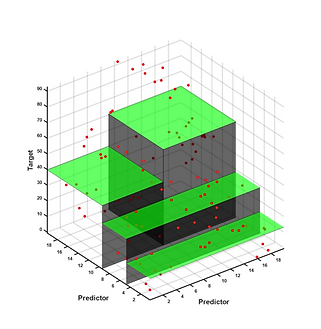
Figure. A newly developed hybrid global optimization algorithm which uses multi-threads competition strategy from various types of evolutionary algorithms
Research Project: Multi-objective Optimization and Multi-criteria Decision Making
Background:
-
In real world, there are many goals could not be met simultaneously. The decision making for water resources management and allocation is also a multi-criteria goal achieving process, which is challenging from both theory and application perspectives.
-
A classical approach to solving a multi-objective optimization problem is to create a new composite function by giving individual weight to each single-objective function and adding them together. Then, the new weighted objective function is optimized using classical gradient-based methods or direct searching schemes. This is a very straightforward approach.
-
However, the fundamental differences between multi-objective optimization and single-objective optimization are ignored when using the transformation of many single-objective functions into a weighted composite function.
Approach:
-
We use the concept of Pareto Optimality and further applied to water and environmental decision-making process.
-
One of the fundamental differences between multi-objective optimization and single-objective is the existence of trade-offs among different competitive objective functions. In other words, for any multi-objective optimization problem, any gain with respect to the fitness of one objective function requires a sacrifice of the fitness in another objective function.
-
We develop different multi-objective optimization techniques algorithms, which are useful and applicable to a broad spectrum of engineering and science problems.

Figure. An Example for a Two Objectives Minimization Problem